An Overview of the US Healthcare market
US healthcare is massive. At $4T, it comprises 40% of the global healthcare market and has grown by 2X over the last decade. The US spends 20% of its GDP on healthcare. In comparison, economies like France and Germany spend 10-12%, while other large economies like India and China spend 4-6%.
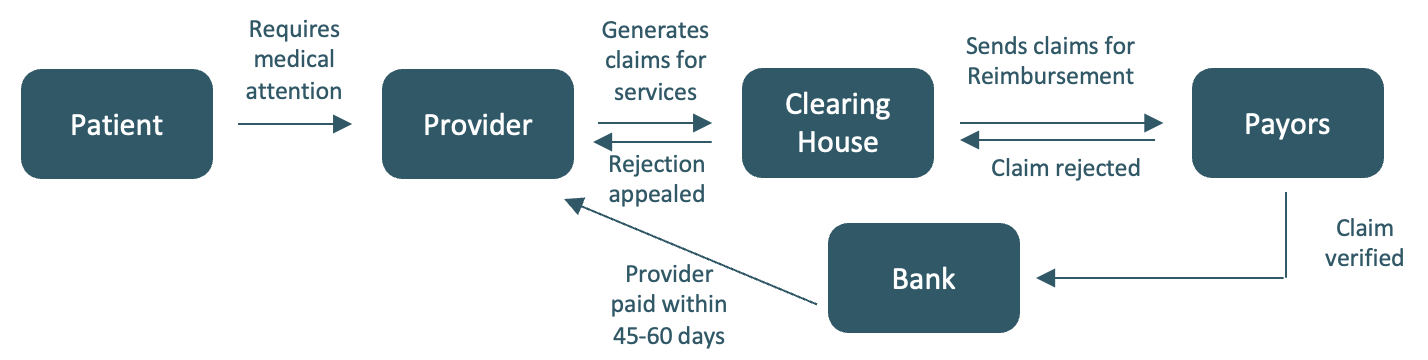
The US healthcare market is unique, characterized by a highly inefficient payor-provider structure. Each year, the US healthcare system spends $1T YoY on admin infra to manage this complex flow of money. In 2022 alone, providers ranging from large hospitals to small physician groups cumulatively spent $450B (~50% of overall spends) on admin spends.
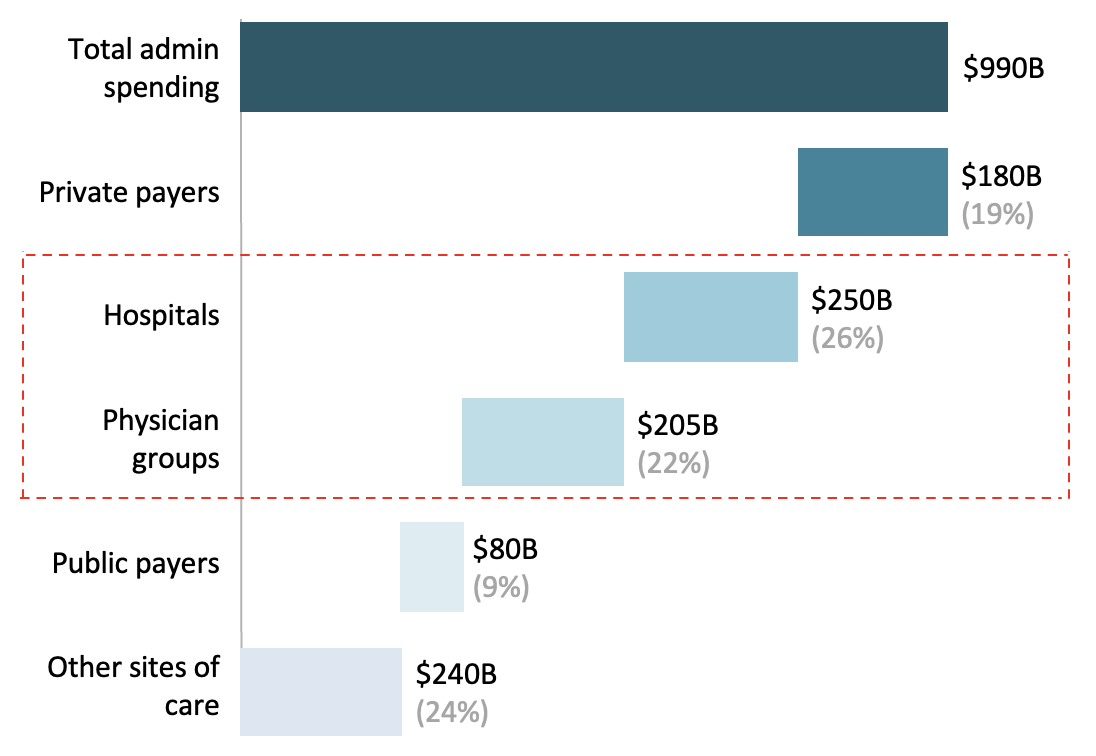
Despite such high spends, estimates place denied claim volumes at $200-300B (nearly 10% of total claims filed by providers). Even post-appeal, $100-150B worth of claims are rejected overall. In other words, after providing $100-150B worth of medical services YoY, providers are not paid due to a range of administrative inefficiencies ranging from improper medical coding to missing or invalid claim data.
The Revenue Cycle Management (RCM) industry, estimated at $120-150B (2023) is geared toward solving for this trillion dollar inefficiency. Of the 100+ healthcare unicorns around the world, 10 are partially or fully focused on the US RCM market and for good reason. Implementing RCM tools across the value-chain (patient scheduling to claims management) can save medical providers ~$400B by 2028.
Revenue Cycle Management
The Revenue Cycle Management market is comprised of multiple pieces that correspond to the flow of money from patient to payor to provider. Some of the key components of this market are as follows:
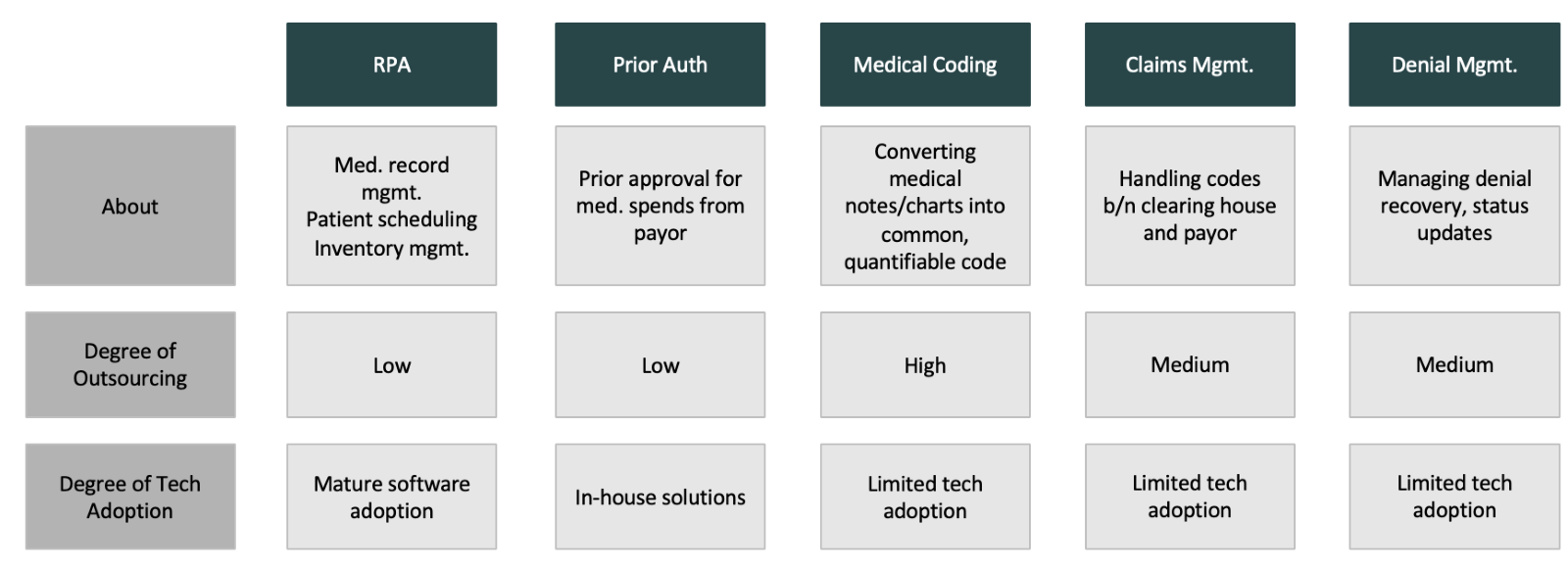
Robotic Process Automation
This is the backbone of the health admin market; everything from managing patient scheduling, inventory and medical record management.
Companies like Olive and Akasa have been solving for the broken hospital backend market for many years.
Prior Auth
Every patient in the US must get prior approval from their insurance company for a medical cost they are about to incur. If the insurer does not cover a particular procedure, providers are not re-imbursed for their services. Improper prior-auth contributes to >7% of overall reasons for claim denials.
Companies like Rhyme are solving for this by reading through patient insurance policies to prevent defaults and improper approvals.
Medical Coding
Every service provider must have a patient’s medical chart converted into a medical code (7-digit alphanumeric code issued by the government). These codes are sent to the payor. Basis the accuracy of the code provided, providers are re-imbursed for their services. If the code is inaccurate, payors do not reimburse the provider - irrespective of the value of the service provided.
This is a big problem and multiple players including Fathom, Nym and CodaMetrix are solving for the same.
Claims and Denial Management
Claims generated by providers are sent to clearing houses which in turn send it to the payors. Managing thousands of receipts, big and small, is no small feat and requires extensive infra to manage. Further, as mentioned before, >$100B worth of these claims are denied each year due to a variety of reasons.
Sending out claims, raising and managing appeals for denied claims etc. is an extensive task and is handled by companies like Candid Health, Adonis, Enter etc.
It’s clear that the US revenue cycle management space is vast and complex. In this article, we will be deep-diving into medical coding - a vast, automate-able market witnessing a clear “why-now” moment because of the recent advances in Generative AI.
What is medical coding?
Imagine you are 50 year old, sitting alone at home with a monitor in front of you and a few large directories on your desk. These directories are filled with alphanumeric codes - each code ascribed to a specific procedure or test available across hospitals and clinics in the US. You are tasked with reading a patient’s medical charts and identifying the relevant code that matches the charts. It takes anywhere between 5 minutes to generate a simple code (basic check-up, doctor prescribed some anti-allergens) to over 2 hours for complex codes (patient spent a week in the hospital). You need to convert 50-70 of these charts into codes every day. Every year, you need to acquaint yourself to a new coding directory afresh and you are paid under $60K/year for your effort.
If this sounds overwhelming and dull at the same time, you are beginning to understand why this profession is dwindling around the world, and why soon an ageing coding workforce will transition out of the job entirely.
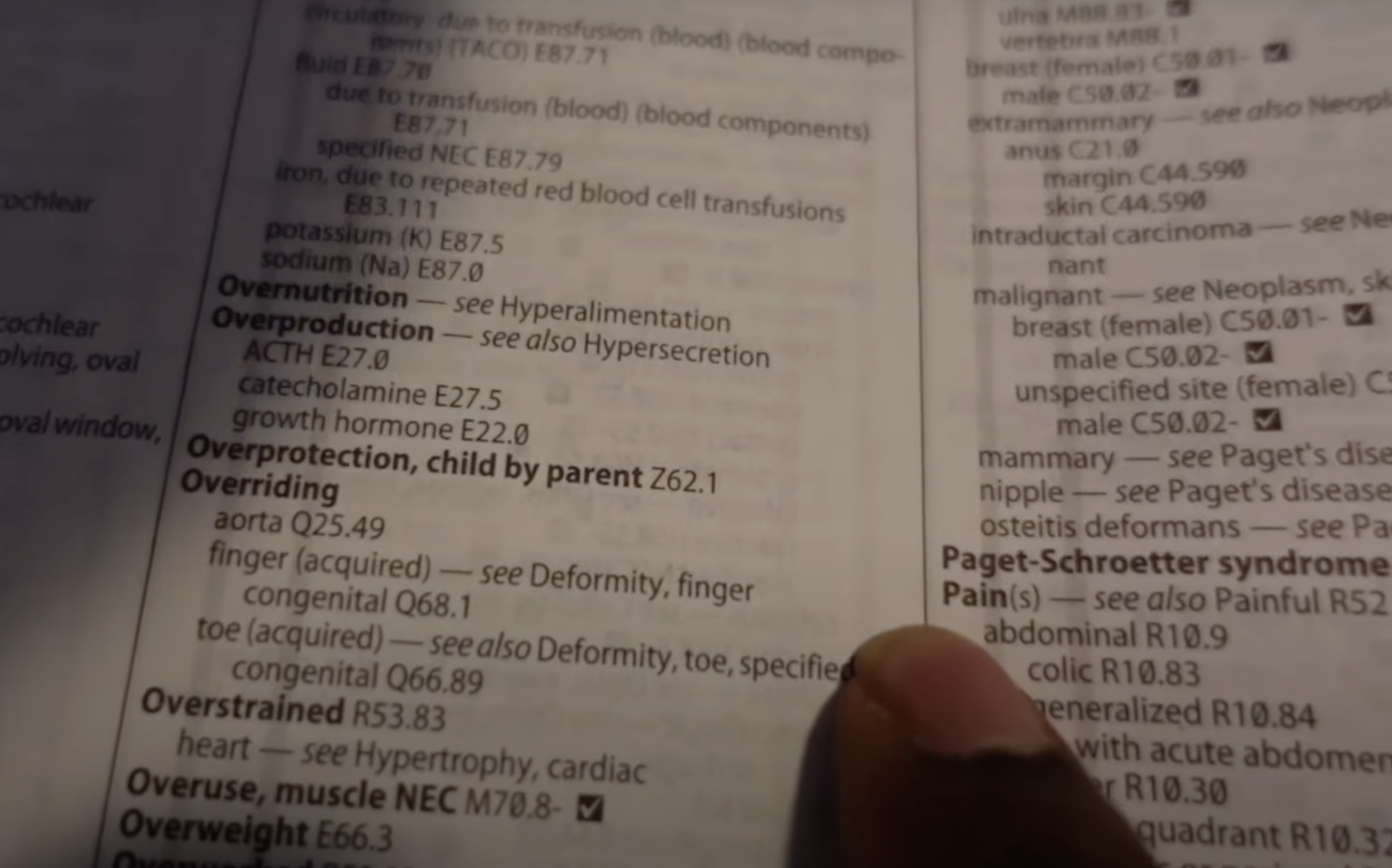
Despite the strikingly unremarkable nature of this profession, it is the backbone of US healthcare. Any medical provider in the states is reimbursed for their services only if the medical code generated against the chart is accurate and approved by the payor. If the code is inaccurate, providers don’t get paid. Period. This $15B market currently hinges on a medical coder’s ability to convert charts into codes - quickly and accurately.
There is a clear opportunity to automate this process and build efficiencies in the way providers create codes in order to send to payors. While computer-assist plays have existed in this market for a while now, (Optum, 3M etc. have product suites enabling human coders to accomplish their task faster), recent breakthroughs in generative AI have created an unprecedented opportunity to fast-track this process like never before.
Enter the AI
Automation is not new - far from it. Over the past few years, players like Nym, Fathom and CodaMetrix have been training their models to convert medical charts into codes with no human assistance. They’ve raised substantial amounts of capital and have achieved a great degree of accuracy, with minimal human intervention, in converting the charts into codes. While Nym and Fathom have origins in out-patient departments (OPD) like radiology and the emergency, CodaMetrix is focused on in-patient departments (IPD) like surgeries.
There are two dominant tech-forms used to train these models. Computational linguistics or a rule-based engine, identifies existing gaps in coding against a benchmarked set of codes. This method requires lesser data and the output is explainable (which means there is an audit trail that explains the origin of the code). Deep learning or a replication engine, does the opposite - it generates an output basis existing data to generate the ‘best-possible’ one. This is a black-box approach (no trail explaining the origin of the code) and such models require a high degree of data to become functional.
Latest LMs have created a third bowl of porridge - one that combines advantages of both traditional systems. The model is iterative, quick to ramp-up and trains on significantly lesser data.
The most important metric for automated coding is accuracy of the code generated. Despite being slow and expensive, human coders achieve ~92-93% accuracy across OPD and IPD. Any automated solution performing under 80% is effectively redundant. With the latest LMs, not only can the model perform at par to human coders in OPD, but also achieve >80% in IPD.
Things to consider when building
We believe founders foraying into this market can adopt the following playbook to win the medical coding market:
Specialties
Founders building in this space should target an OPD specialty like radiology. Given the relatively standardised mechanism of coding, the accuracy is higher and allows for time and data to train the model. Today, Nym and Fathom operate in the radiology segment.
While OPD specialties act as the GTM, the real prize pool lies in accurately coding IPD specialties at par to human coder accuracy. Given the reams of charts created in IPD, automation accuracy typically falls under 80%. Training models on this data is also tricky given a lot of this data is proprietary and requires partnerships with large hospitals in the US to gain access. Founders that are able to build accurate automated coding solutions for IPD specialties leading to better CFs and fewer denials, will likely win this market.
GTM
Cracking partnerships with large hospitals and health systems is the pathway to achieving PMF and scale. Selling to CFOs of mid-market hospitals or directors of revenue cycle management at large hospitals/health systems can create inroads to lasting relationships and a data moat that is hard to crack.
While small physician groups can also be targeted, the ACVs are lower and data-wins small. However, they are an attractive ICP for long-term market capture.
What’s the India right-to-win?
India has been at the forefront of RCM innovation for many years. Chennai and Hyderabad are big coding hubs and Indian SaaS sales professionals have had years of experience selling software to US healthcare. With AI, founders can take India’s involvement and leverage in this market to a whole new level.
Indian founders building in this space can win along three core vectors. They are as follows:
Product
India is a thriving hub of coding talent - much of the outsourced coding work happens in large coding companies spread across the country. Partnering with these companies to build out the MVP and launching the product to their clients provides in-roads into an otherwise opaque industry.
GTM
India is rich with healthcare native talent with decades of experience selling software to US hospitals. An in-domain team with deep networks in US healthcare can greatly assist in solving for the initial journey and cracking the first set of large contracts that will catapult the company into PMF.
Cost
Despite the accuracy levels achieved by automated coding solutions, a human-in-loop will always be required for high-value claims where error can lead to millions in damages for providers. Such a product plus service solution will find a natural fitment in India where the cost arbitrage and availability of talented and tenured coders is high.
Risks
Despite the bright outlook, some key challenges of building in this space must be noted.
Automated code accuracy – Any automated coding solution is only as good as the output’s accuracy. Products that require a high-degree of human-in-loop will not scale. Founders building in this space will have to ensure their models are trained to an extent where the output is either at par with human coder efficiency or displaying a clear pathway to achieve similar accuracy levels.
GTM – The entire sales motion is in the US, which means that a team building from India will have to sell effectively to the target ICP there. Given existing competition and the time-consuming process of converting large enterprise clients, founders will face headwinds in achieving PMF and premium logos.
We would love to chat.
At Matrix Partners India, we are very bullish on Indian founders building AI-first products to solve for the massive RCM market. In our next piece, we will cover other parts of the RCM stack and discuss how AI can disrupt these industries. If you’re building in this market, drop us a line at health@matrixpartners.in.